Abstract: A novel deep studying technique that makes use of graph convolutional neural networks (gCNNs) can predict cognitive operate primarily based on the mind’s dimension and construction. The algorithm could present insights into the connection between mind morphology and totally different cognitive features, in addition to declines in cognitive operate.
Supply: Northwestern College
Northwestern investigators have developed a deep learning-based technique that may predict cognitive operate capability primarily based on mind form and construction, detailed in a research revealed in Scientific Reviews.
The tactic, which makes use of graph convolutional neural networks (gCNNs), might also reveal new insights into the connection between mind morphology and totally different cognitive features in addition to the decline of mind operate.
“After we apply the wealthy capabilities of CNNs to graph illustration of the mind, we are able to discover the mind as a picture in a beforehand unexplored approach,” stated S. Kathleen Bandt, MD, assistant professor of Neurological Surgical procedure and a co-author of the research.
Understanding how the connection between mind construction and cognitive operate modifications all through the life course has remained elusive. Nevertheless, earlier work means that fluid intelligence—the flexibility to drawback clear up and suppose and cause abstractly—relies upon closely on two areas of the mind: the prefrontal cortex and parietal cortex, each of that are concerned in decision-making and sensory notion, amongst different features.
Moreover, investigating the affiliation between mind construction and cognitive operate could present extra details about mind maturation and ageing, in addition to the physiological causes of cognitive impairment.
Within the present research, the investigators developed novel gCNNs, a specialised deep studying mannequin that extracts distinct morphological options resembling cortical thickness and subcortical construction from transformed mind MRI scans to foretell fluid intelligence.
“We’re decreasing the mind to its surfaces solely, that means that we’re in a position to seize data not solely about folding patterns, but in addition about curvature and relationships between surfaces throughout tens of hundreds of nodes together with the outer cortical floor, the internal cortical floor and the floor of seven subcortical mind constructions,” Bandt stated.
Utilizing their novel gCNNs, the scientists extracted morphologic data of cortical ribbons and subcortical constructions from two giant MRI datasets involving sufferers from totally different age teams.
Utilizing this strategy, the investigators had been in a position to exhibit that their mannequin considerably outperformed different related state-of-the-art strategies and that utilizing a mix of cortical and subcortical constructions offered essentially the most correct predictions.
Moreover, throughout each datasets, they discovered that structural options of the amygdala, hippocampus and nucleus accumbens (NAc), together with temporal, parietal and cingulate cortex, drove the fluid intelligence prediction.
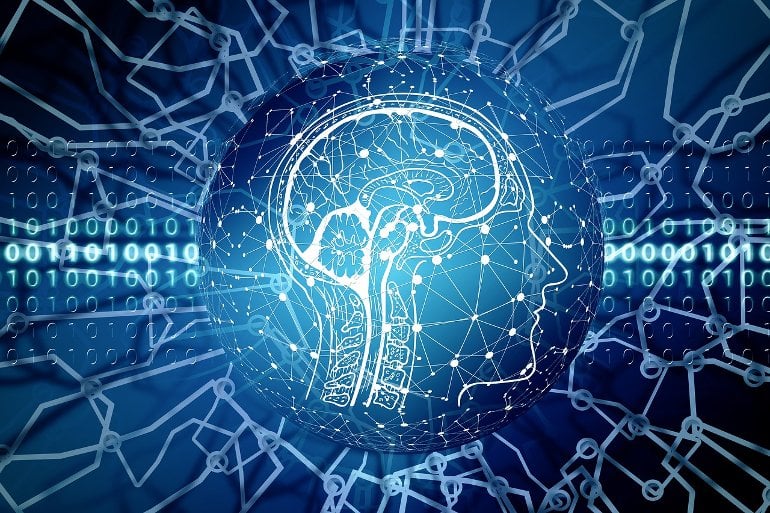
“Prior work investigating the neuroanatomic substrate of fluid intelligence has recognized associations between widespread cortical areas, however comparatively few relationships have been reported with subcortical constructions.
“Our research added to those research by figuring out involvement of the bilateral NAc, hippocampus within the prediction of fluid intelligence, which have been linked to the features of cognitive science resembling reward processing in judgment and decision-making in addition to emotion regulation,” stated Yunan Wu, Ph.D., a graduate scholar within the McCormick Faculty of Engineering’s Division of Electrical and Pc Engineering and lead writer of the research.
In keeping with the authors, their surface-based gCNNs supply the chance to map recognized relationships between neurocognition and mind anatomy for myriad analysis functions. The tactic additionally requires much less coaching time and computations, making it extra environment friendly to use to different complete datasets.
For instance, one other latest research led by Bandt used gCNN evaluation for ageing and dementia, discovering that the tempo of mind ageing differs between wholesome people and sufferers with dementia.
“We at the moment are seeking to see if related cognitive measures will be predicted utilizing this technique as has been completed in our work right here on fluid intelligence, but in addition can we predict the onset of dementia and doubtlessly forestall or delay it earlier than it begins,” Bandt stated.
About this deep studying and cognition analysis information
Creator: Melissa Rohman
Supply: Northwestern College
Contact: Melissa Rohman – Northwestern College
Picture: The picture is within the public area
Authentic Analysis: Open entry.
“A multicohort geometric deep studying research of age dependent cortical and subcortical morphologic interactions for fluid intelligence prediction” by Yunan Wu et al. Scientific Reviews
Summary
A multicohort geometric deep studying research of age dependent cortical and subcortical morphologic interactions for fluid intelligence prediction
The connection of human mind construction to cognitive operate is advanced, and the way this relationship differs between childhood and maturity is poorly understood.
One sturdy speculation suggests the cognitive operate of Fluid Intelligence (Gf) relies on prefrontal cortex and parietal cortex.
On this work, we developed a novel graph convolutional neural networks (gCNNs) for the evaluation of localized anatomic form and prediction of Gf. Morphologic data of the cortical ribbons and subcortical constructions was extracted from T1-weighted MRIs inside two unbiased cohorts, the Adolescent Mind Cognitive Growth Research (ABCD; age: 9.93 ± 0.62 years) of youngsters and the Human Connectome Undertaking (HCP; age: 28.81 ± 3.70 years).
Prediction combining cortical and subcortical surfaces collectively yielded the very best accuracy of Gf for each ABCD (R = 0.314) and HCP datasets (R = 0.454), outperforming the state-of-the-art prediction of Gf from some other mind measures within the literature.
Throughout each datasets, the morphology of the amygdala, hippocampus, and nucleus accumbens, together with temporal, parietal and cingulate cortex persistently drove the prediction of Gf, suggesting a major reframing of the connection between mind morphology and Gf to incorporate methods concerned with reward/aversion processing, judgment and decision-making, motivation, and emotion.